Challenges to data integrity in the pharma lab
To prioritize data integrity in pharmaceutical R&D, companies must implement strong data practices throughout the process, ensuring the fidelity and confidentiality of all data types, including raw data, metadata, and transformed data. This involves addressing challenges related to data governance and security, especially as organizations digitize data for scalable analytics. Evolving data management strategies is critical to mitigating risks, as data integrity violations can impact research validity, patient safety, and product efficacy.
The increasing complexity of R&D processes and technologies, coupled with multimodal R&D across global locations, creates challenges in maintaining data integrity. Collaboration among research groups is essential for efficient data sharing, but differences in data management practices complicate efforts. Standardizing these practices, as recommended by guidelines like the FAIR principles, can improve data findability, accessibility, and reusability.
Artificial intelligence (AI) is becoming integral to R&D, necessitating updated data infrastructures to handle growth in data volumes, eliminate silos, and ensure compliance with global regulations, such as the EU's Artificial Intelligence Act. This aims to protect health, safety, and fundamental rights as AI is increasingly applied in research.
Ultimately, good data practices are key to aligning data management with regulatory and ethical standards, supporting long-term research success, and preparing for the future of automated and collaborative research. Modern tools help scientists manage, search, and visualize R&D data, with future solutions focusing on integrating these applications within secure platforms.
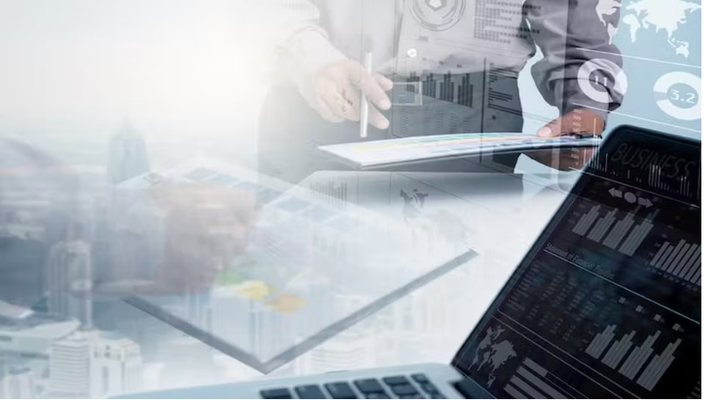
Comments
No comments posted yet.