Scientific papers
The pharmaceutical industry is increasingly adopting innovative, data-driven tools to enhance, optimize, and accelerate the drug development process. Extended-release (ER) oral formulations offer numerous benefits, such as maintaining therapeutic drug levels, reducing dosing frequency, and minimizing side effects. However, achieving consistent drug release profiles remains a significant challenge. In vitro dissolution testing, a key factor for drug absorption into systemic circulation, is a complex and time-intensive process used to evaluate these formulations.
This study aimed to develop a predictive model for drug dissolution in the quality by design (QbD)-based development of ER hydrophilic matrix tablets containing polyethylene oxide (PEO). Two modeling approaches were explored and compared: (i) screening multiple machine learning (ML) models to identify and deploy the most effective model—artificial neural networks (ANN) in this case—and (ii) applying functional data analysis (FDA) combined with the design of experiments (DoE) to fit a smoothing model to each dissolution curve as a continuous function.
A dataset of 91 ER matrix tablet formulations was analyzed, with dissolution data divided into training (70%), validation (20%), and test (10%) sets. Both the ANN and functional DoE (FDOE) models demonstrated high similarity to experimental dissolution profiles, as reflected by f2 values ranging from 48 to 88 for FDOE and 52 to 88 for ANN.
This study underscores the potential of integrating advanced data-driven modeling techniques into ER drug development. By improving dissolution prediction accuracy and streamlining the formulation process, these approaches can significantly reduce development time and costs.
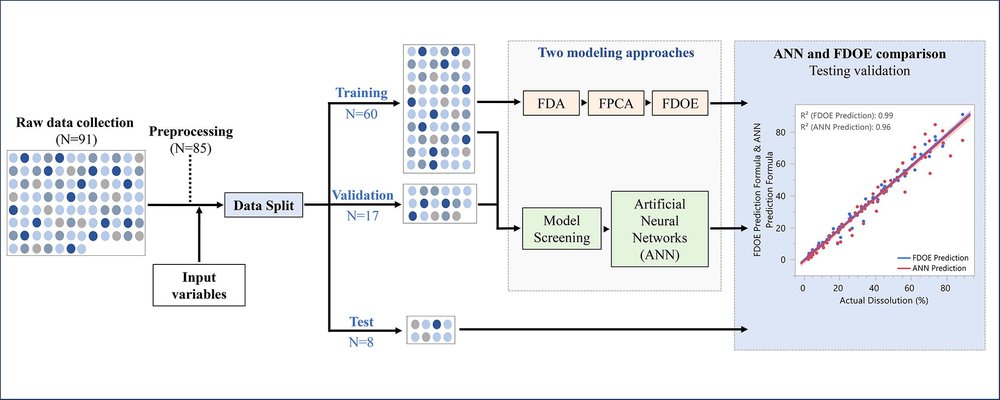
Comments
No comments posted yet.
Add a comment