Scientific papers
T-shaped partial least squares regression (T-PLSR) is an effective machine learning technique for developing formulations and manufacturing processes for new drug products. Building an accurate T-PLSR model requires experimental data across various formulations and process conditions. However, collecting extensive experimental data with large-scale manufacturing equipment is often difficult due to high costs, time constraints, and significant raw material consumption. This study introduces a hybrid modeling approach combining T-PLSR with transfer learning (TL) to improve the predictive performance of a T-PLSR model for large-scale manufacturing data by utilizing a substantial amount of small-scale manufacturing data. The T-PLSR+TL method was applied in a case study focused on scaling up the tableting process from a well-established compaction simulator to a less-experienced rotary tablet press. The T-PLSR+TL models showed significantly improved prediction performance for tablet quality attributes of new drug products compared to T-PLSR models that did not use large-scale manufacturing data for new drug products. The findings demonstrated that T-PLSR+TL is more effective in addressing new drug products by using small-scale manufacturing data to compensate for the lack of large-scale manufacturing data. Additionally, T-PLSR+TL has the potential to streamline formulation and manufacturing process development for new drug products using a comprehensive database.
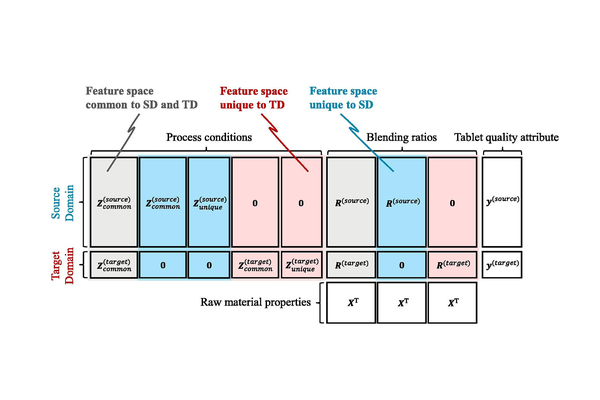
Comments
No comments posted yet.
Add a comment