Scientific papers
Utilizing both machine learning (ML) techniques and physics-based modeling, this study introduces two feature-based reduced-order models. One model is designed for the quantitative prediction of density, while the other is tailored for the classification of the diametrical hardness of pellets produced through a powder compaction process (pelleting). To ensure interpretability, these models exclusively utilize input parameters derived from a modified Drucker-Prager Cap (DPC) model, calculated from process data monitoring and the maximum applied compression force.
For quantitative density prediction, eight features associated with first-principles models of powder compaction are generated, with the final model utilizing only two. A crucial aspect of the modeling, and a significant contribution, involves a data augmentation step. This step leverages smaller supplementary data sets, enriching the primary data set and incorporating measurements not present initially.
The outcomes indicate a noteworthy reduction in the quantity of data required for model input, thereby decreasing the costs associated with data acquisition, storage, and computational time. Additionally, a detailed analysis of the impact and relevance of the generated features on model performance is provided. The density prediction model, using just two features, achieves a mean absolute scaled error (MASE) of 12.9% and a mean absolute error (MAE) of 0.10 (where r2 = 0.975). The scaled (diametrical) hardness classifier exhibits an F1 score of 0.915 with the use of four features.
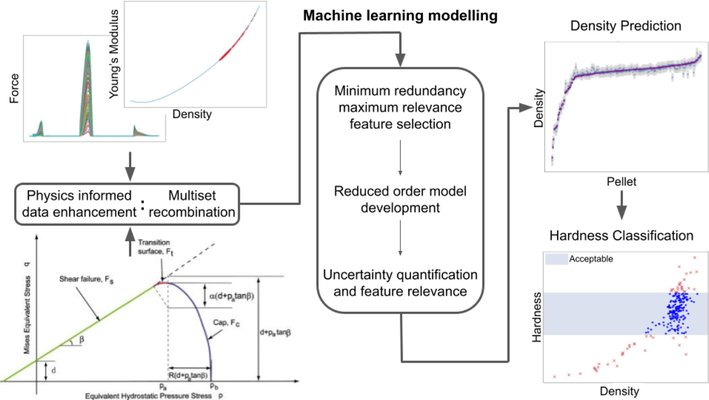
Comments
No comments posted yet.
Add a comment